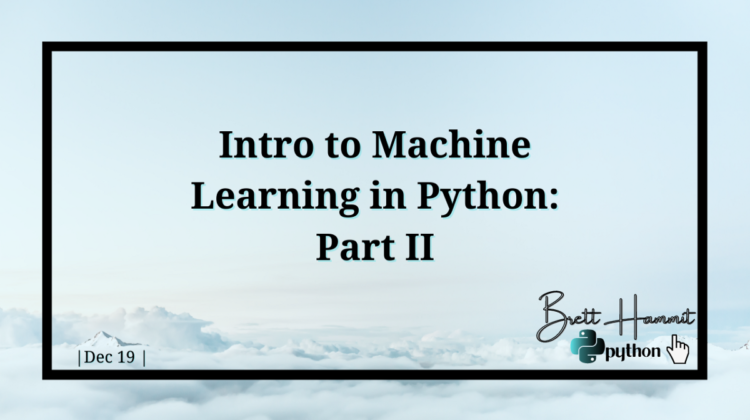
- Machine Learning Summary
- Splitting the Data
- Training and Testing Data
- Creating and Training the Model
- Wrapped Up
In Intro to Machine Learning in Python: Part I we talked about what linear regression is, how to analyze the data that we have and then how to plot out some visualizations to give us a better understanding of our data and the correlations between it. In this post we will be going through what machine learning is in basic terms, as well as how to split, train, test data and fit our models. Let’s hop right into it!
To sum machine learning up simply you could say that we feed AI large amounts of data and it uses statistics to make predictions based upon the data that we give it. This is achieved with the different variety of algorithm’s that we discussed in the first post. Machine learning is used in a variety of different ways and one example of this is betting sites using machine learning models to set the lines for different bets making them as accurate as possible.
The steps of machine learning normally go with first gathering data, cleaning the data, putting majority amount of data in training data, and then the rest into test data, from there we go into model testing and finish with deploying the model into real world applications. Visually looking like this:
Now that we have an idea of what machine learning is we can get into how to do the first step in linear regression which is splitting the data up.
Like I talked about in Part I I am using the scikit-learn package within Python. So in order to be able to split the data we need to first set the data into x and y arrays. The y array will contain what we are trying to predict and the x array will have all the other non-text data in our data frame. Linear regression will not work with text data unless for natural language processing which I am looking to get into after machine learning. You might want to get a good view at all of your column names so to make this easier I just make a call for a sorted list to view all of them. To do all this in code it would look like this:
sorted(df)y=df['ColName']
X=df['ColName','ColName','ColName','ColName']
Now that we have split the data into their appropriate arrays we can go about training and testing our data.
To train and test our data it is very simple where we just need to import the train_test_split method from the scikit learn package. After doing this we are going to test and the train the data looking like this:
from sklearn.model_selection import train_test_splitX_train, X_test, y_train, y_test = train_test_split(X, y, test_size=0.3, random_state=101)
This is making sure that we are training and testing both data in the x and the y array as well. The test_size parameter is the amount of data being put into the test data. Normally 30 percent goes into test data and 70 percent goes into training data, but this can be subject to change based on the model. To clear things up maybe a bit for example in the code above we are giving .3 out of 1.0 so that means .7 will go into the training data. The random_state parameter is the number of random splits in our data.
We have now successfully split the data, and then trained and tested it. The next step is to start training our model!
In order to create and train the model we will:
- import linear regression from sklearn
- cast linear regression to lm to make it easier for us to call
- fit the the model while passing it our training data
Doing these tasks the code will look like:
from sklearn.linear_model import LinearRegressionlm = LinearRegression()
lm.fit(X_train,y_train)
What fitting the model does is we give our model the data so that it specifically knows the context of what it is working with and what tools to use. After fitting it shows the estimator parameters of the machine learning algorithm and what they are set to. These can be changed to fine tune the model that you are making. This link will take you to the documentation of scikit-learn’s linear regression if you want to really fine tune your model or learn more about the depths of linear regression.
Congrats! We have now done all the framework that goes into creating a linear regression model! To go over what we learned: what machine learning is, how to split train and test data, and finally how to create and fit a linear regression model! Now that we have created our model we need to analyze and evaluate the model that we made which will be shared in Part III of this series!
Thank you for taking the time out to read this and if you enjoyed it drop a like or a comment telling me what you liked or disliked or with any questions or topics that you might wanna hear about next!