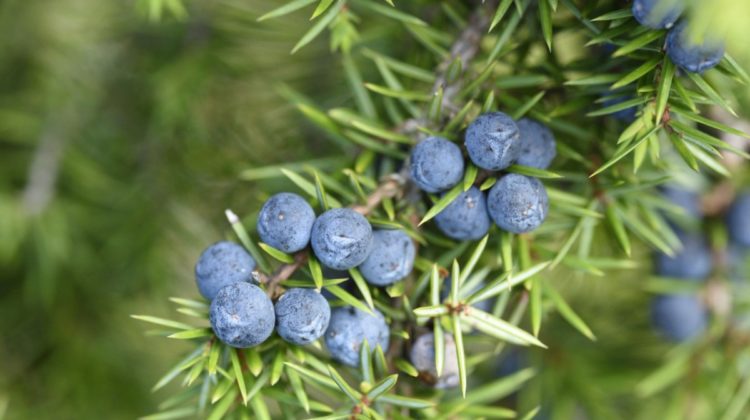
As you can see there is clearly a relationship between a patients age and their depression score. Now what if we want to describe this relationship objectively? I think we can agree that the relationship looks visually linear, but the exact equation of this linear relationship is unknown. In real life, it may be useful to predict a new patients depression score based on some key demographics, such as age. This may help a general practitioner screen many patients quickly to ascertain patients at a higher risk of depression and consult these patients with a degree of priority. In order to make this prediction, we need to know the equation of the line that fits the data best. Obviously, this is an oversimplification for comprehension, but stay with me.
The first key part of this problem is that we assume the relationship between our input data and our outcome data is linear. This is one of the 4 major assumptions required by linear regression and is not always as straight forward as one might think. If the relationship between our data points looks more complex than a linear descriptor (y = mx +c) then perhaps a different tool should be used.
Linear relationship → This means for a unit increase in X, we have a proportional unit increase in Y.
So, we are happy that our relationship can be assumed to be linear, what next? Well now we need to decide where our “line of best fit” should go. We could decide this by trial and error. This would involve drawing many lines, such as the line below and visually inspecting which lines looks better.