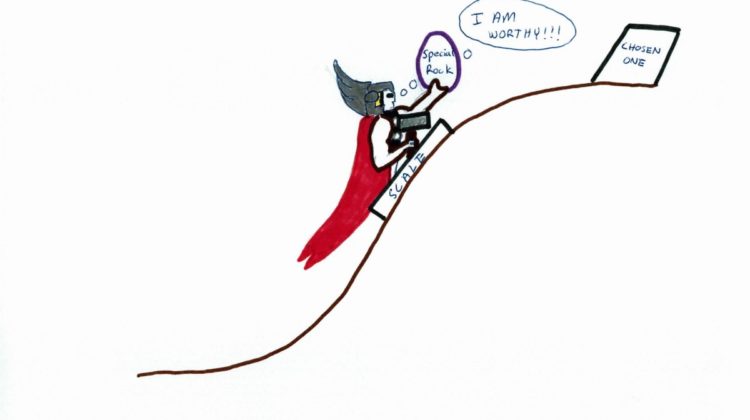

‘Logistic Regression’ is the appropriate regression analysis to conduct when the dependent variable is dichotomous (binary). Source: Statistics Solution
For the conceptual overview of Logistic Regression, refer — A Comprehensive Guide to Logistic Regression
We shall now go through the code walkthrough for the implementation of the logistic regression algorithm from scratch:
import numpy as npclass LogisticRegression:
def __init__(self, learning_rate=0.001, n_iters=1000):
self.lr = learning_rate
self.n_iters = n_iters
self.weights = None
self.bias = Nonedef fit(self, X, y):
n_samples, n_features = X.shape# init parameters
self.weights = np.zeros(n_features)
self.bias = 0# gradient descent
for _ in range(self.n_iters):
# approximate y with linear combination of weights and x, plus bias
linear_model = np.dot(X, self.weights) + self.bias
# apply sigmoid function
y_predicted = self._sigmoid(linear_model)# compute gradients
dw = (1 / n_samples) * np.dot(X.T, (y_predicted - y))
db = (1 / n_samples) * np.sum(y_predicted - y)
# update parameters
self.weights -= self.lr * dw
self.bias -= self.lr * dbdef predict(self, X):
linear_model = np.dot(X, self.weights) + self.bias
y_predicted = self._sigmoid(linear_model)
y_predicted_cls = [1 if i > 0.5 else 0 for i in y_predicted]
return np.array(y_predicted_cls)def _sigmoid(self, x):
from sklearn import datasets
return 1 / (1 + np.exp(-x))
from sklearn.model_selection import train_test_splitdef accuracy(y_true, y_pred):
accuracy = np.sum(y_true == y_pred) / len(y_true)
return accuracybc = datasets.load_breast_cancer()
X, y = bc.data, bc.targetX_train, X_test, y_train, y_test = train_test_split(X, y, test_size=0.20, random_state=1234)
regressor = LogisticRegression(learning_rate=0.0001, n_iters=1000)
regressor.fit(X_train, y_train)
predictions = regressor.predict(X_train)
accuracy(y_train, predictions)Out:
0.9298245614035088predictions = regressor.predict(X_test)
accuracy(y_test, predictions)Out:
0.9186813186813186
Hope this helps! Good Luck 🙂
For complete code implementation:
To contact, or for further queries, feel free to drop a mail at — tp6145@bennett.edu.in