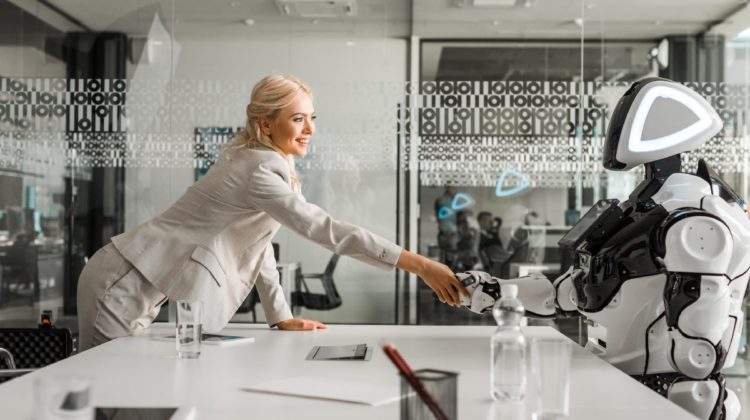
The last year was quite intense for me, as together with my partners — I successfully raised Pre-seed funding for our startup — AGICortex. And believe me, it was not an easy task to convince people that we will change how Artificial Intelligence is done forever while having in hand only results of our research and some proof-of-concept demos.
But now, in 2021 when we are slowly coming out of the shadows — we believe in it even more. Let me share some of our findings that led to defining our company’s long-term strategy: using local data processing (edge devices) in static analytical machines, then using gained awareness about the physical environment in autonomous machines: drones, robots & vehicles to make them much better than they are today. In the next steps, we have plans for some cloud-based products, that will also use the understanding of the physical world gained in the previous steps.
This unusual sequence of steps will allow us to get to the level of human-like Artificial General Intelligence in the next couple of years.
The AI agents and solutions of the future will be native to both the physical and digital world.
Sounds interesting? Then read this compressed summary of what we have learned in 2020 and the previous years:
It is important to realize that as humanity, we have collectively created much more incorrect than genuine knowledge. Only to be improved by the next generations.
Just think about how people understood the world in the past (e.g. Earth as the center of the Universe), how we treated diseases (leeches therapy anyone?), or the deep belief of many past generations that the world is built with solid matter and not moving energy particles.
The same is with our inventions and tools. We have replaced post pigeons with telegraphs, phones, and then a global computer network called the Internet.
Is this the ultimate medium of communication?
I highly doubt and to be honest, it is naive to think that what is now will be like that in the next centuries.
There were people claiming that we already discovered everything important in physics or that there is no reason for an individual to have a personal computer…
You know how it ended.
We like to think about ourselves, our species, or just our generation as special and unique. It is in our nature.
But the objective reality tells us that everything changes all the time.
It is not reasonable to think that future technologies will be strictly based on Deep Learning. Even if it achieved so many successes — and today there is nothing more sophisticated that simulates true intelligence.
Just like it doesn’t make sense to assume that what you or I work on today, will be the ultimate solution to the problems we decided to solve. It is just the way it is.
But it is so crucial to make the next step again and again. What humanity needs to progress with AI are automated knowledge creators, not data-hungry artificial statisticians. Let me explain it more below.
How it can be that we may need weeks of training to possess some skills, but acquire knowledge immediately if it is presented in a useful form?
You can recognize someone’s face after seeing his photo only once. You can learn some historical facts just after you will read about them in a book.
It is also possible to understand the idea after hearing the clear explanation.
Somebody may try to explain to you how it is to ride a bike or drive a car — but you need to try it for yourself multiple times to master that skill.
Only complex skills require many repetitions with many corrections to achieve satisfying results.
On the other hand, you can immediately use the acquired knowledge and it is very easy to update it based on new recent experiences or information.
Is not this a clue for how to build more effective AI systems?
The fact that something works well, doesn’t mean there is nothing better.
Knowledge is much easily transferred than skills between different people (or in future artificial agents) because of its universal nature.
Data (raw) ➔ Information (classifications) ➔ Explanation (model of relations) ➔ Knowledge (future applications)
Because of differences in attributes of bodies (humans) and components (machines) and its complexity – the case of skills is a bit harder and requires more adaptation in a sequence of learning iterations.
Also, sensory processing paths may learn immediately and gradually improve the accuracy, when needed. These conclusions just beg for hybrid neuro-symbolic architectures combining artificial neural networks with graph-like structures.
Then, some basic skill hypotheses may be transferred among machines to be verified in practice and adapted. Just as you can’t master the skill just by watching a training video or reading some tips — but it can shorten the required time by pointing you in the right direction.
In the human brain, we have a structure called the cerebellum („the little brain”) that has the largest density of neurons — containing 75–80% of the total amount in only 10% of its volume.
We may also simplify the situation a bit and state that cerebrum (the largest part with two hemispheres) is active more in our conscious processes, where the cerebellum leads the unconscious processes like movement and keeping posture.
The digital equivalent of the cerebellum would be a perfect place to store the proven & verified models of skills, that require little to zero further optimization. Instead offering incredibly fast execution.
On the other hand, the part mimicking the real neocortex and the cerebrum would be responsible for active learning during the process of acquiring more data through experience.
With a large group of highly capable autonomous AI knowledge creators, we could significantly accelerate the process of acquiring and verifying new knowledge about not only previously unreachable places (space, oceans) but also more familiar places, where they would simply see what we could not recognize before.
Artificial statisticians that need carefully prepared examples and constant human supervision are not the optimal solution here. The intellectual and creative force on Earth may be multiplied only with a fully autonomous technology.
As humans, we all have a lot of cognitive biases. (This infographic lists 188 of them if you are curious to check…)
„Confirmation bias is the tendency to search for, interpret, favor, and recall information in a way that confirms or supports one’s prior beliefs or values.”
There is no wonder that if someone devoted his life to something — he will continue to believe in its most crucial foundations. It would just be preferred to leave things as they are now.
Also, the companies that have successful business models relying on specific mechanisms — like sending all data to the cloud for processing and making better ad profiling solutions at the same time — will not be so willing to change them.
The market for IT professionals is shaped by the needs of companies. The graduates are equipped with knowledge demanded by those organizations or shared by their lecturers.
Change happens slowly…
I would prefer solutions that privately process all my data and therefore may have richer access to it. The environmental cost of the most complex Deep Learning models also starts to become significant. Not to mention the lack of transparency and a requirement to hire a whole team of IT professionals to maintain the solutions.
I am impressed by our technical progress in the last decade with deep respect to the hard work of all researchers and inventors, but for sure it is not the ultimate state.
That is why questioning everything may be one of the most important skills to teach (as soon as possible) yourself, your children… or your Artificial Intelligence systems.
They need to be able to recognize exceptions to the rule on their own.
They need to be able to imagine things that do not yet exist. And not always to seek for a good enough solution — but the best one.
Let’s have the similar courage to make the next step after the technology that has its foundations in the 80s of the previous century.
Just like Deep Learning models are in most cases obscure to the human eye, the progress of Artificial Intelligence towards AGI will be visible to fewer and fewer people.
And not only because of the increased complexity of the technology or science behind it. But due to the requirement of merging knowledge from the different disciplines, e.g. neuroscience, biochemistry, mathematics, software development & hardware design.
For example, Computational Neuroscience has different goals than Artificial Intelligence — by modeling brain activities in a much more precise way.
But I believe that both sides may learn from each other — and taking more inspiration from the human brain may lead to progress in AI. And the opposite — progress with technology may result in a better understanding of our brains and ourselves.
Let’s not stop there, why not add biochemistry, psychology, and other science disciplines?
There is a high risk of failure if we will just stick to mathematically optimized models of neural networks, without a true understanding of who we are and what our bodies are doing…
That is why some people may state that we have still 50–100 years to experience advanced AGI. Or that we can’t digitize emotions (what is not true), so machines could understand them.
While I believe that we may experience AGI in this decade, by having enough understanding of what the brain does and why — not exactly how. The technology does not need to reflect all processes, including those that are designed to grow and sustain a biological organ.
At AGICortex we already have a detailed plan of software and hardware development that leads to exactly this goal. And we are going to realize it.
The emergence of AGI may shock people that did not see it as a sequence of consecutive steps. The answers are available to those of us who are curious enough to ask the right questions.
Our eyes have different types of receptors:
- rods — used to see at a very low level of illumination or at night, very sensitive but color blind
- L-cones, M-cones, S-cones: that respond to the wavelengths of different sizes and therefore different colors (red, green, blue respectively)
Besides the explanation of daltonism, this fact shows us how adaptable creatures we are. Our vision systems are not only based on color recognition. We can see in poor lighting conditions too.
We have this duality of two eyes, ears, hands, legs, brain hemispheres, etc. And it leads to more capabilities, in terms of vision — it contributes to the perception of depth. For ears — it allows for better location of the sound sources.
The structure of our brain allows processing multiple data types together in a very efficient way. But also its prioritization — what is a more salient visual feature at the moment. Is it something that is in the move or closer to us?
We know that humans are not perfect. But we are so adaptable and designed to be autonomous and survive in so many conditions.
It is a true miracle of biotechnology to be inspired by.
So now imagine that you can combine various sensors: depth or infrared cameras, microphone arrays, and even radio frequency sensors allowing you to see through walls…
But you are limited with your software that can’t combine motion sensing with the mechanism of detecting visual attributes for faster high-definition video stream processing.
We need to build AI systems that will be able to work with multiple data types efficiently in a single neural architecture.
Because it increases accuracy, makes the learning faster, optimizes energy expenditures on processing (e.g. don’t process the part that was not changed from previous frames).
There are sensors and software solutions that can give true superpowers to our AI while keeping it fully autonomous.
Just like ensemble models that are successful in many tasks — we can use multiple channels of input data classification to respond in the most optimal way.
Neuroscience — even the name suggests what is the main subject. Neurons. But is it true?
Glial cells have long been regarded as mere fillers, just supporting neural activity. But we have more and more proofs that it is exactly the opposite.
- Radial progenitor cells — provide the necessary initial structure for the brain
- Astrocytes — control the excitatory and inhibitory synapses, using neurons as fast electric wiring while leaving a significant role of master conductors for themselves
- Microglia — protect the brain from dangers as a part of its immune system, but also contribute to adult brain plasticity, removing the unnecessary synaptic connections
Suddenly with glial cells, we have much more options to be inspired by the brain and think about possible algorithms.
Introducing some structure to the brain allows for creating separate, but cooperating modules for different data types — just as the brain has regions responsible for seeing, hearing, other senses, and movement.
The astrocytes may collect additional information about the neural activity that we could compare to the meta-data — and I can imagine multiple scenarios of how it may be useful in neural architectures. Some have already been implemented in our innovative 3D neural networks.
Also removing less relevant parts leads to making the whole system more efficient and accurate.
This structure powered by meta-data can allow not only for incremental learning without a separate training phase, but also the creation of more complex neural units and utilization of additional modules to route the data to the right places and recombine it for new tasks.
Not bad for a mere filler…
I have heard somewhere that AI still waits for its Steve Jobs — and that mass adoption will require front-end simplicity combined with back-end complexity.
I could not agree more. That is why as an independent researcher I always tried to work on solutions that we will be able to offer to regular non-tech people. And I continue this approach at our company.
Forget about command-line scripts and complicated tools. These are good for the developers. The future will belong to those that will offer seamless integration with our digital and physical environments.
Just like we will have multiple sensors in our homes and offices — we will want to quickly interact with our AI systems from multiple locations through convenient audiovisual interfaces.
Think about current smart assistants combined with visual input/output mechanisms delivered through smart mirrors, 3D holographic screens or whatever method we will prefer in the future — but strongly integrated with the look of our home or office.
The mirror will change into the screen when you will need this.
You will be able to see explanations about how AI makes its decisions and what data its stores upon request made in the most convenient for you method. If 3D visualization is better, then we will have it presented like that. Such screens are already on the market.
But as AI will be more and more integrated with our daily lives, having rich access to our data and working autonomously — we will demand two things:
Privacy and to remain in control — by a clear understanding of how our data is collected and used for our benefit.
This is not something that can be provided by the current state of technology. We will need full transparency about the internal operations delivered in an understandable form — not a complex post-fact attempt to explain how the system worked.
That is why, at AGICortex — we work on visual management tools for AI, that provide full transparency about our 3D neural networks.
Obviously, there will be many scenarios where we will still use centralized cloud-based data processing — for others often local data processing will be preferred. Especially when the result benefits a single individual or a small group of people in the same location.
For privacy, reduced latency, accessibility and the environment.
The biggest brains of the next decades will no longer work on surveillance systems and serving you better ads ➔ they will deliver powerful AI solutions to machines at your homes & offices.
With the further development of local (Edge AI) processing units — most of the use case scenarios will be fine without the cloud.
And this doesn’t mean that we will not share any learnings between the devices. It just means that we will not need a justification for collecting sensitive data about our private lives in the data centers.
Highly accurate AI systems will require more data about us. And we will prefer to process it locally.
At the same time, collected meta-data in innovative neural architectures may allow for rapid optimizations of specific features.
Some of us may be excited by 5G/6G networks, but the latency associated with sending data to the cloud and waiting for a response may be too high for some types of machines.
There is a reason why the data should be kept close to the processing unit — the ‘time cost’ of its transportation can be thousands of times higher in physically distant units.
In the case of autonomous drones, robots & vehicles that difference may mean the ability to avoid some serious accidents.
The movement to the edge will also mean the development of energy-efficient algorithms that will be able to run on local devices. This will increase accessibility and will also benefit the environment.
Sounds really cool, but how actually do all these things described above?
The neuro-symbolic architecture of 3D neural networks with supporting modules, designed by AGICortex was intended to form a digital equivalent of the human brain.
Of course, right now we work on smaller tasks like static machines for audiovisual AI assistants. And only in the next phases, we will equip them with more ways to respond to the input data — through their motion components.
Nevertheless, this approach provides energy-efficiency, explainability, modularity, compatibility with multiple data types that are required by autonomous Artificial General Intelligence. While preserving privacy of the user and offering convenient interfaces of interaction.
The data in neural networks no longer needs to flow only from left to right or right to left. It can go in multiple directions, by being routed via dedicated supporting modules. It allows us to use only those parts of the whole architecture that are useful for the specific task.
It is very similar to how the human brain operates.
Ultimately, we will be able to have massive neural architectures working locally in machines.
I think it is a much better perspective than spending the amount of electricity used by a large city just to train & run a single huge Deep Learning model that tries to provide the capacity of the human brain just by its size and not agility.
I would like to share more about 3D neural networks but:
- it is much better to see them in action than to read a description
- we are working on more detailed materials, as you can imagine the topic is quite complex
- we will launch our first products on the market this year
- we aim to offer them as a part of complete ML framework for physical devices
Stay tuned for more information.
And by the way — if you feel that you have what it takes (lots of determination and curiosity) to be a part of this journey — do not hesitate to contact me.
Maybe we can make it together.