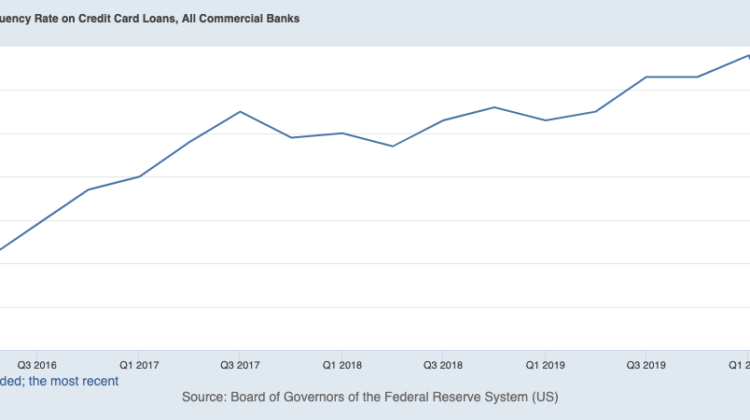
Exploratory Data Analysis
The following are some key findings in the data. Shout to Gabriel Preda from Kaggle for the awesome visualization ideas. Check out his work here:
Distribution of target classes is highly imbalanced, non-defaults far outnumber defaults. This is common in these datasets since most people pay credit cards on time (assuming there isn’t an economic crisis).
Payment status. Correlation strength increases the closer the months are in time. Makes sense. For example, one could assume a late payment in August would likely lead to a late payment in September. However, it is less clear we can make the same assumption for April and September
Distribution of credit limit amounts. The three largest credit limit amount groups are $50k, $20k, and $30k, respectively.
Credit Limit by Sex. The data is evenly distributed amongst males and females.
Marriage, age, and sex. The dataset mostly contains couples in their mid-30s to mid-40s and single people in their mid-20s to early-30s.