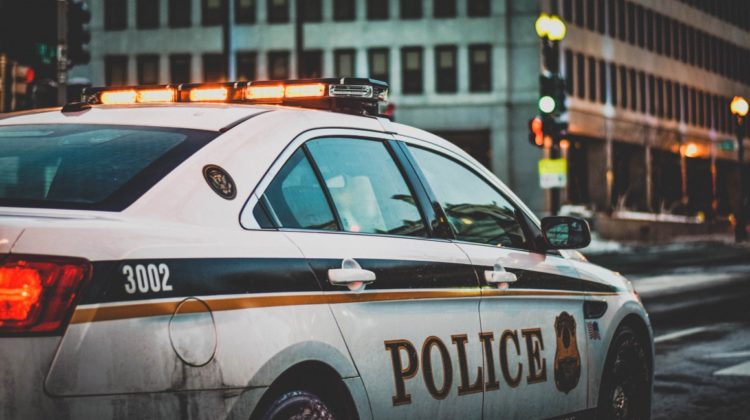
Imagine sending your 12 years old child to buy some milk at the supermarket near your house. You know that your neighbourhood is safe and you let him go alone. Unfortunately, something happens.
A bad guy approaches your child trying to get him in his car: your child is about to be kidnapped. At the same moment, a patrol approaches and two police officers hear your child screaming.
They get out of their car and they arrest the bad guy.
Your child is safe.
Wow! How lucky are you that a patrol was there in that exact moment, isn’t it?
Well, that was not lucky. It was predictive policing.
There is not a unique definition of what predictive policing means.
The idea behind it is to use analytical techniques to identify targets for police intervention and prevent crime.
In this article, I would like to draw your attention to the evidence available today about the effectiveness of predictive policing, as well as the major issues linked to the usage of such technologies.
The most used software in the US in 2020, is called PredPol. Developed by a UCLA professor, PredPol is designed to predict when and where a specific crime will occur in the next 12 hours. With daily updated data, PredPol displays predictions using color-coded boxes on a map.
This software, like Keystats, HunchLab, Palantir and others, uses the so-called hotspot analysis to identify locations of statistically significant hot spots and cold spots, based on the data given.
An example of a different technique used is KeyCrime. KeyCrime, developed by an italian police officer, uses the crime linking technique with the idea of finding the connection between a series of crimes and then predicting when and where the same criminals are most likely to hit next.
The key point here is that, whatever technique the software uses, the main goal is to provide insights that allow to better deploy police officers such that they can be in the right place at the right time. It’s a matter of resource allocation to maximize efficiency.
The city of Santa Cruz, California was one of the first cities in the country to adopt predictive policing technologies back in 2011. After nine years though, Santa Cruz became one of the first cities in the U.S. to approve a ban on its use.¹
In 2020 the Los Angeles Police Department, one of the oldest customers of PredPol, announced the stop of the predictive-policing program because of “financial constraints caused by the coronavirus outbreak”. The problem was not only financial: they have not been able to meaningfully evaluate the program’s overall effectiveness.²
Those are just examples, but predictive policing is still used in many cities across the United States.³
The actual effectiveness of predictive policing cannot be evaluated universally, because it depends on the model used and should be evaluated individually. A literature review made in 2019, found that predictive policing technologies have potential but not all crimes can be reduced through them.
As an example, since the implementation of predictive-policing technologies, the overall crime index decreased by 6% in New York.⁴
In other places though, there are not such relevant results that can justify a transition from traditional methods.
Putting financial reasons and effectiveness aside, the biggest concern around those technologies is that they can lead to inequalities between social groups and racism.
Algorithms trained with arrest data can be biased because police officers in the U.S. are known to arrest more Black people or people in other minority groups. Also, arresting someone doesn’t mean that he or she will be convicted. On the other hand, training algorithms on the victim’s report is problematic as well, because of the likelihood to report a crime.⁵
Victim reporting is also related to community trust or distrust of police. So if you are in a community with a historically corrupt or notoriously racially biased police department, that will affect how and whether people report crime.⁵
Rashida Richardson — Lawyer and researcher who studies algorithmic bias
Furthermore, there is a growing concern regarding a lack of transparency on how these algorithms work and why they return certain outcomes. To protect their business, vendors do not want to share these kinds of information and it is difficult to work with black boxes because, when they are wrong, it’s hard to find out how to fix them.
To put it in another way, if a police officer takes a bad decision, he can explain why. If the police officer makes a bad decision because of a suggestion made by the algorithm, he cannot explain anything other than:
“the algorithm said that”