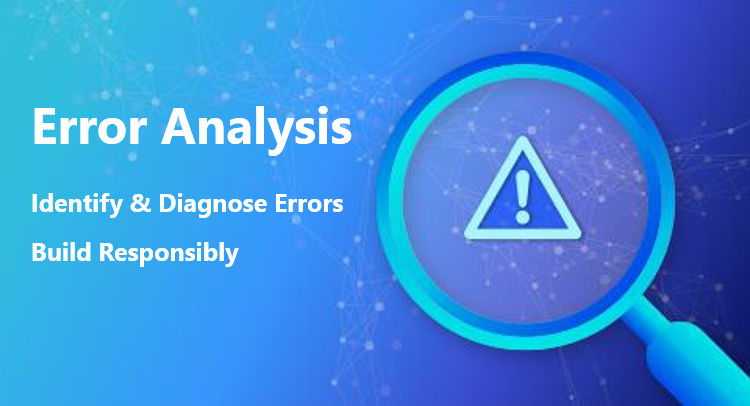
Error Analysis starts with identifying the cohorts of data with a higher error rate versus the overall benchmark error rate. The dashboard allows for error exploration by using either an error heatmap or a decision tree guided by errors.
Error Heatmap for Error Identification
The view slices the data based on a one- or two-dimensional grid of input features. Users can choose the input features of interest for analysis. The heatmap visualizes cells with higher error with a darker red color to bring the user’s attention to regions with high error discrepancy. This is beneficial especially when the error themes are different in different partitions, which happens frequently in practice. In this error identification view, the analysis is highly guided by the users and their knowledge or hypotheses of what features might be most important for understanding failure.
Decision Tree for Error Identification
Very often, error patterns may be complex and involve more than one or two features. Therefore, it may be difficult for developers to explore all possible combinations of features to discover hidden data pockets with critical failure. To alleviate the burden, the binary tree visualization automatically partitions the benchmark data into interpretable subgroups, which have unexpectedly high or low error rates. In other words, the tree leverages the input features to maximally separate model error from success. For each node defining a data subgroup, users can investigate the following information:
- Error rate — a portion of instances in the node for which the model is incorrect. This is shown through the intensity of the red color.
- Error coverage — a portion of all errors that fall into the node. This is shown through the fill rate of the node.
- Data representation — number of instances in the node. This is shown through the thickness of the incoming edge to the node along with the actual total number of instances in the node.
Cohort definition and manipulation
To specialize the analysis and allow for deep dives, both error identification views can be generated for any data cohort and not only for the whole benchmark. Cohorts are subgroups of data that the user may choose to save for later use if they wish to come back to those cohorts for future investigation. They can be defined and manipulated interactively either from the heatmap or the tree. They can also be carried over to the next diagnostical views on data exploration and model explanations.
After identifying cohorts with higher error rates, Error Analysis enables debugging and exploring these cohorts further. It is then possible to gain deeper insights about the model or the data through data exploration and model interpretability.
Data Explorer: Users can explore dataset statistics and distributions by selecting different features and estimators along the two axes of the data explorer. They can further compare the subgroup data stats with other subgroups or the overall benchmark data. This view can for instance uncover if certain cohorts are underrepresented or if their feature distribution is significantly different from the overall data, hinting therefore to the potential existence of outliers or unusual covariate shift.
Instance views: Beyond data statistics, sometimes it is useful to merely just observe the raw data along with labels in a tabular or tile form. Instance views provide this functionality and divide the instances into correct and incorrect tabs. By eyeballing the data, the developer can identify potential issues related to missing features or label noise.
Model interpretability is a powerful means for extracting knowledge on how a model works. To extract this knowledge, Error Analysis relies on Microsoft’s InterpretML dashboard and library. The library is a prominent contribution in ML interpretability lead by Rich Caruana, Paul Koch, Harsha Nori, and Sam Jenkins.
Global explanations
Feature Importance: Users can explore the top K important features that impact the overall model predictions (a.k.a. global explanation) for a selected subgroup of data or cohort. They can also compare feature importance values for different cohorts side by side. The information on feature importance or the ordering is useful for understanding whether the model is leveraging features that are necessary for the prediction or whether it is relying on spurious correlations. By contrasting explanations that are specific to the cohort with those for the whole benchmark, it is possible to understand whether the model behaves differently or in an unusual way for the selected cohort.
Dependence Plot: Users can see the relationship between the values of the selected feature to its corresponding feature importance values. This shows them how values of the selected feature impact model prediction.
Local explanations
Global explanations approximate the overall model behavior. For focusing the debugging process on a given data instance, users can select any individual data points (with correct or incorrect predictions) from the tabular instance view to explore their local feature importance values (local explanation) and individual conditional expectation (ICE) plots.
Local Feature Importance: Users can investigate the top K (configurable K) important features for an individual prediction. Helps illustrate the local behavior of the underlying model on a specific data point.
Individual Conditional Expectation (ICE): Users can investigate how changing a feature value from a minimum value to a maximum value impacts the prediction on the selected data instance.
Perturbation Exploration (what-if analysis): Users can apply changes to feature values of the selected data point and observe resulting changes to the prediction. They can save their hypothetical what-if data points for further comparisons with other what-if or original data points.
Error Analysis enables practitioners to identify and diagnose error patterns. The integration with model interpretability techniques testifies to the joint power of providing such tools together as part of the same platform. We are actively working towards integrating further considerations into the model assessment experience such as fairness and inclusion (via FairLearn) as well as backward compatibility during updates (via BackwardCompatibilityML).
The initial work on error analysis started with research investigations on methodologies for in-depth understanding and explanation of Machine Learning failures. Besmira Nushi, Ece Kamar, and Eric Horvitz at Microsoft Research are leading these efforts and continue to innovate with new techniques for debugging ML models. In the past two years, our team was extended via a collaboration with the RAI tooling team in the Azure Machine Learning group as well as the Analysis Platform team in Microsoft Mixed Reality. The Analysis Platform team has invested several years of engineering work in building internal infrastructure and now we are making these efforts available to the community as open source as part of the Azure Machine Learning ecosystem. The RAI tooling team consists of Ilya Matiach, Mehrnoosh Sameki, Roman Lutz, Richard Edgar, Hyemi Song, Minsoo Thigpen, and Anup Shirgaonkar. They are passionate about democratizing Responsible AI and have several years of experience in shipping such tools for the community with previous examples on FairLearn, InterpretML Dashboard etc. We also received generous help and expertise along the way from our partners at Microsoft Aether Committee and Microsoft Mixed Reality: Parham Mohadjer, Paul Koch, Xavier Fernandes, and Juan Lema. All marketing initiatives, including the presentation of this blog, were coordinated by Thuy Nguyen.
Big thanks to everyone who made this possible!
Towards Accountable AI: Hybrid Human-Machine Analyses for Characterizing System Failure. Besmira Nushi, Ece Kamar, Eric Horvitz; HCOMP 2018. pdf
Software Engineering for Machine Learning: A Case Study. Saleema Amershi, Andrew Begel, Christian Bird, Rob DeLine, Harald Gall, Ece Kamar, Nachiappan Nagappan, Besmira Nushi, Thomas Zimmermann; ICSE 2019. pdf
Updates in Human-AI Teams: Understanding and Addressing the Performance/Compatibility Tradeoff. Gagan Bansal, Besmira Nushi, Ece Kamar, Daniel S Weld, Walter S Lasecki, Eric Horvitz; AAAI 2019. pdf
An Empirical Analysis of Backward Compatibility in Machine Learning Systems. Megha Srivastava, Besmira Nushi, Ece Kamar, Shital Shah, Eric Horvitz; KDD 2020. pdf
Understanding Failures of Deep Networks via Robust Feature Extraction. Sahil Singla, Besmira Nushi, Shital Shah, Ece Kamar, Eric Horvitz. CVPR 2021 (to appear). pdf