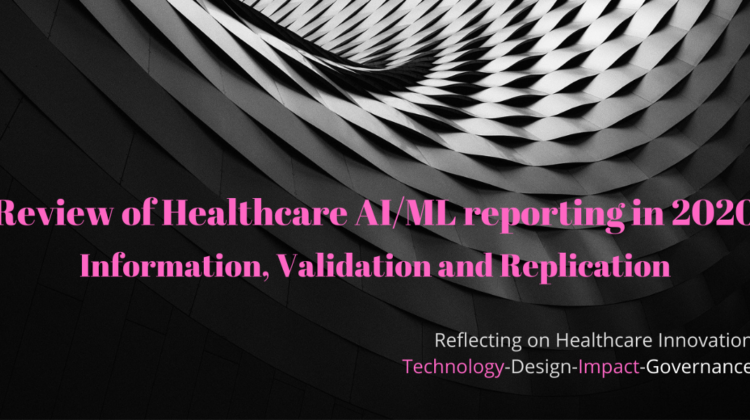
A recap on ‘how to read healthcare publications about AI/ML’
This reading guide written by Liu et al in the Journal of American Medicine gives a good overview of what to look out for in any literature source that uses machine learning methodology. These can be categorized broadly in 4 separate categories, with each category helping us understand different facets of the quality of research-
- Machine learning methodology : How is the specific method chosen? Why was it chosen? Are these questions addressed at all in the article?
- Data sources and quality: What are the data sources? Is this representational of the disease area? If not, has this been noted in the article as a limitation? How did the researchers handle missing data? Was there a chance of overfitting due to any steps of data preparation?
- Results and performance: What kind of performance evaluations are described? Are the results unexpected or look too good? Does the article describe independent validation, possibly in a prospective study?
- Clinical implications: how will such a ML Model will be implemented in real world clinical setting? how will the clinical effect be measured and monitored?
As you can see each of these topics will give us better understanding about the methodology used and thereby help us read the article in a critical manner.
Accompanying this fantastic guide was a commentary by Finale Doshi-Valez & Roy H Perlis-Evaluating Machine Learning Articles ²
Doshi-Valez and Perlis reiterate the point about the basis for using machine learning-underlying assumptions, model properties, optimization, strategies, and limitations. It is necessary to understand the data sources and regularization techniques used to validate the results.
They also add a few more considerations-
- Subgroups: There has been already enough debate about inherent racial and gender bias elsewhere in algorithmic world including healthcare algorithms. Due to the complexity of algorithms, it might be possible to have hidden systematic errors and hence any research literature that provides detailed analyses of results across different subgroups will be essential to understand training parameters.
- Larger may not be better: Due to inherent biases in data sources as well as data preparation techniques used, we will need to be careful not to equate large validation sets with better models.
- Clinical Setting: There needs to be more intense scrutiny for models that start as retrospective studies and imply use in a prospective manner in clinical setting. It will be important to understand which features are driving the results and how are they linked to our known clinical/medical knowledge before we start applying them in real-world setting.
For a good primer on definitions of commonly used performance evaluation parameters , refer to this detailed primer (by M Yu et al) on different measures commonly used in such literature.