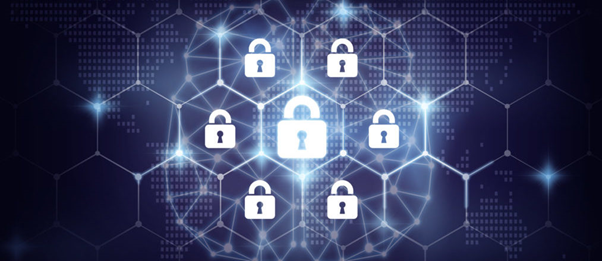

With AI / ML having a boom, aptly supported by the robust Cloud ecosystem which has sprung up in the last 18~24 months many organizations are in the early stages of adopting and integrating ML infrastructure with their solutions. Organizations are seeing immense value in traversing this path as they see this as a future in the post pandemic world. Having said that , It is equally important to keep Security and Data privacy in mind while transforming a regular business solution to an AI driven solution. Here I present a quick and short outline of the paramount security risk considerations which ought to be mitigated while developing an agile AI/ML based solution.
Data plays a key role in the security and overall quality of an ML system. That’s because an ML system learns to do what it does on the basis of the input data. If an attacker can intentionally manipulate the input data being used by an ML system in a coordinated fashion, the entire system can be compromised, and the results will henceforth become unreliable and incorrect. Data-poisoning attacks require special attention. In particular, ML engineers should consider what fraction of the training data an attacker can control and to what extent.
There are several data sources that are subject to poisoning attacks whereby an attacker intentionally manipulates data, including raw data in the world and datasets that are assembled to train, test, and validate an ML system, possibly in a coordinated fashion, to cause ML training to go awry. In some sense, this is a risk related both to data sensitivity and to the fact that the data themselves carry so much importance in a ML System.
An ML system is said to be “online” when it continues to learn in a real time basis during the operational use, thereby modifying its behavior over time. The intent of this to keep the system evolving with a focus towards improvement. In this case a clever attacker can trick the still-learning system in the wrong direction on purpose through system input and slowly & gradually “retrain” the ML system to do the wrong thing. Note that such an attack can be both subtle and reasonably easy to carry out. This risk is complex, demanding that ML engineers consider data provenance, algorithm choice, and system operations in order to properly address it.
Also known as Transfer Learning Attacks , many ML systems are constructed by tuning an already trained base model so that its somewhat generic capabilities are fine-tuned with a round of specialized training. This takes the advantages of reusable design . A transfer attack presents an important risk in this situation. In cases where the pretrained model is widely available, an attacker may be able to devise attacks using it that will be robust enough to succeed against your (unavailable to the attacker) tuned task-specific model. The knowledge of base model , especially if it is in public domain gives ample opportunity to the attacker to try out various attack scenarios and best optimize the attack plan against you system. You should also consider whether the ML system you are fine-tuning could possibly be a Trojan that includes sneaky ML behavior that is unanticipated.
ML systems are re-used intentionally in transfer situations. The risk of transfer outside of intended use applies. It is generally expected for the authors / organizations which posting models for transfer would do well to precisely describe exactly what their systems do and how they control the risks in an well elaborated articulated outline.
Data protection is difficult enough without throwing ML into the mix. One unique challenge in ML is protecting sensitive or confidential data that, through training, are built right into a model. Subtle but effective extraction attacks against an ML system’s data are an important category of risk.
Preserving data confidentiality in an ML system is more challenging than in a standard computing situation. That’s because an ML system that is trained up on confidential or sensitive data will have some aspects of those data built right into it through training. Attacks to extract sensitive and confidential information from ML systems (indirectly through normal use) are well known. Note that even sub-symbolic “feature” extraction may be useful since that can be used to hone adversarial input attacks. It is therefore recommended to enforce Data at rest protection controls which includes things like encryption , access control and role based data access .
Setting aside the core learning algorithms and data the other key part of any AI / ML based solution is the underlying application and infrastructure on which it is hosted. This brings to the forefront the standard application security practices that needs to be put in place in essence addressing the OWASP Top 10 security issues is a good starting point. It is also worth the effort to have dedicated and devoted threat modelling session around the application design and hosting .
The underlying infrastructure should be secure by design and care should be taken to secure any kind of API Keys or shared secret in use. Besides this the configuration settings and endpoint security (Securing data in motion / AuthN & AuthZ ) are the other key areas .