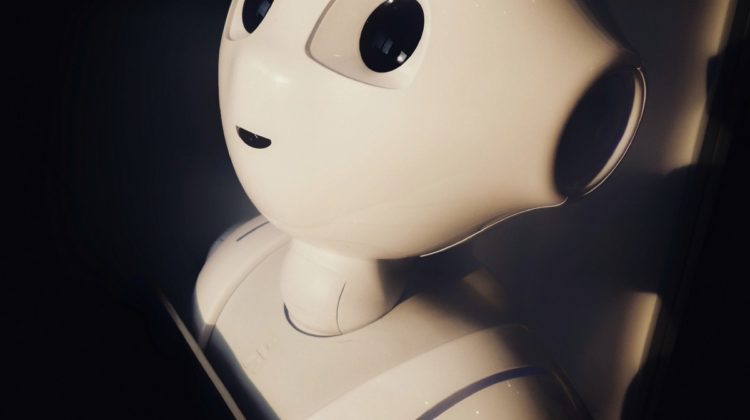
“Don’t apply to PhD programs in ML. It’s just too competitive.”

As far as Silicon Valley goes, “machine learning” has become the buzzword of the day. From first-year computer science students to industry professionals, people across tech are fascinated by its possibilities.
I’ve seen this obsession firsthand at UC Berkeley, where I’m finishing my Bachelor’s in Applied Math. Students who have little interest in pursuing ML take CS 189, Berkeley’s grueling introductory course to the topic, torturing themselves for no clear reason other than the belief that it is somehow necessary for a chance at a job in tech.
I also fell under this spell, convinced that the end goal of studying computer science was to build up the proficiency to understand ML. In the midst of my own fixation on the topic, a rather wise friend said something that stuck with me:
“The amount of people obsessed with getting into machine learning right now scares me. You have all these people who think they’re pursuing a quick path to a high-paying job, but soon it’s going to overflow and anyone who isn’t a complete genius will have nowhere to go.”
The effects of this overflow are already strikingly visible in the realm of academia, and they will soon make their way into industry.
Let’s start with the the state of academia. In much the same way that ML jobs are coveted in industry, ML PhD programs are easily the most competitive programs of study at any university in the country. If you can get into M.I.T., Berkeley, or Carnegie Mellon — and that’s a big if — you’re set for life as an academic researcher.
This begs the question — just how good do you have to be? To find out, I spoke with one of my professors about the reality of applying to ML PhD programs. Most research professors love to encourage students to pursue their fields (it is their life’s work, after all), but not this time.
“Don’t apply to PhD programs in ML. It’s just too competitive.”
When I pressed him for details, he revealed that students applying to PhD programs in ML who get in often have 3–4 published research papers at some of the top academic venues in the country (think NeurIPS). Keep in mind that these are undergraduates — some barely old enough to legally purchase alcohol. And what’s more, if you haven’t published, your application won’t even be considered.
Why does this matter? After all, the proportion of tech people who pursue academia over industry is minuscule, so what bearing does any of this have on the future of ML in industry? We don’t care about coming up with the next amazing mathematical equation for a neural net, we just want Alexa to understand us better, right?
Well, as much as some would like to ignore it, research and industry are inextricably linked, especially when it comes to machine learning. And the bleak prospects of succeeding as an ML researcher foreshadow the future prospects in industry as well.
We’re already at a point where many attractive data science and machine learning jobs require at least a Master’s degree. Meanwhile, countless young computer science students say they want to go into cutting-edge ML but have no intention of studying past their Bachelor’s. They are caught up in the hype and do not realize how high the barrier to entry has already risen.
There are job boards and ads all over the internet making the claim “Silicon Valley desperately needs 500000 professionals in data science and machine learning — and you could be one of them.” What these ads don’t tell you is so many people are being funneled into the field that by the time you finish college, you might need to commit to another two years of school before you’re even considered for the job.
Long story short — the ML industry is following in the footsteps of its academic cousin; very soon, being able to secure even one job will require you to be the absolute best at what you do.
So, what’s the solution? It’s quite simple, really.
We need to stop pretending that machine learning is some kind of panacea that will solve all our tech problems immediately.
There are two main misconceptions we need to address:
- Data scientists spend all their time deploying super cool ML models.
- Within computer science, the only exciting discipline today is machine learning.
Regarding the first point, the job title “data scientist” is seriously over-hyped, and not just for the googly-eyed freshmen among us. Even recent grads who’ve secured data science jobs expect to go in and train models all day, which is wishful thinking at best. In fact, according to a twitter poll conducted in 2019 by data scientist Vicki Boykis, fewer than 5% of respondents claimed to spend the majority of their time on ML models. To the surprise of those uninitiated (which sadly includes most college students pursuing data science jobs), most of the time was spent on cleaning and organizing data.
Moving beyond just data science, we need to stop pretending ML is everything. Some of the greatest advancements in computer science have absolutely zilch to do with training or test data. Think Linux, Git, Sketchpad (if you’re a 1960s lover), multicore, and the iPhone. More generally, mobile development, hardware, human-computer interaction, cloud computing, and countless other computational sub-fields are flourishing, even though they don’t receive the bulk of attention.
We’re reaching a tipping point which we can still prevent. ML may be up-and-coming, but we should stop treating it like the holy grail of programming, because it really isn’t. As things currently stand, it has become a buzzword that people blindly pursue without truly knowing what it is. That’s not good — both for ML and for other disciplines in computer science.
But it isn’t too late — with a change of attitude and marketing, we can eradicate the incessant obsession with machine learning, and avoid a bleak situation where a beautiful field becomes so competitive that no one thinks it worth their time to pursue it. Because not even the best training data in the world will fix that.