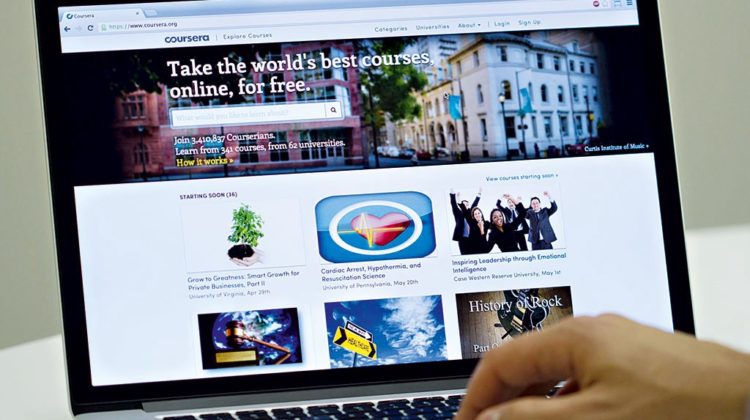

I started the machine learning course with thoughts of ‘Let’s just test my English skills.’, ‘I’m a business student, so I would be satisfied even with learning just some of them.’ However, as I gradually improved, I found machine learning was not only for a genius. Even for students with a non-technical major, it was possible to understand if you have a little bit of mathematical knowledge from high school, though it took some time for me to get used to vector calculation. At this point, studying machine learning from Coursera became more important than my real school work. I crammed my major for the exam and spent the most time studying machine learning.
After completing the machine learning course, I applied to deep learning specialization and started to contemplate having this field as my career. Like the earlier experience with the machine learning course, I was able to get a lot of tangible things out of it. Especially, the system that made me build a code by myself for an assignment to continue to the next lecture was so effective.
However, like there’s no such thing as a perfect course, this course also had some minor problems. One of them was that it didn’t cover a process of preprocessing data as it only focused on deep learning itself. In other words, every data had already been prepared for deep learning models, so there was no opportunity to learn how to deal with data before feeding them to models. Therefore, it would obviously become an obstacle in the future if I don’t know how to do it when I look for a job or implement my projects.
Also, when I think about getting a job rather than just learning, there was quite a bit of confusion from time to time to think, ‘Was I too naive?’, ‘Can I pursue this as a career without a relevant degree?’, ‘I have no one in the IT world around me, who should I ask?’. While I was looking for the answer for them, I got to know Medium website where people from hands-on workers, researchers, to students share their ideas and generate informative content. I learned not only knowledge about deep learning but also, the hype of deep learning, and how to prepare for a journey to get a job. It was an oasis for me who was eagerly looking for such information. The most important thing that I got from them was recognizing the meaning and roles of both data science and data scientist. This made me realize what I should pursue and also answered most questions I got, so I decided to be a data scientist, not a machine learning engineer that I was dreaming vaguely.
Then what’s the difference between a machine learning engineer and a data scientist? A simple analogy can help understand. Let’s say data science is cooking. Then a machine learning engineer is a person who studies only recipes for cooking, so they don’t pay too much attention to where to buy and how to prepare the ingredients. Instead, they focus on how to improve its taste. Therefore a machine learning engineer should be highly specialized and is required to have high academic degrees. On the other hand, a data scientist is one who does all the work from shopping grocery to cooking with already prepared recipes. So, they are not required to be specialized as much as machine learning engineers but should have a broad knowledge to be all-around over the whole process. In terms of technologies, machine learning engineers focus on architecture development that requires highly advanced mathematic knowledge, so they are closer to researchers or academics. On the other hand, data scientists tend to use models already developed by machine learning engineers to solve industrial problems, so they are closer to industry.
As I didn’t have any degrees related to those things, I thought I’d better be a data scientist than a machine learning engineer since I could make use of my domain knowledge. So, I was able to fine-tune my directions for the future and upcoming projects accordingly.
Korean version: 찰리의 늦둥이 블로그 :: 평범한 경영대 학생이 데이터 사이언스를 시작한 이유 — 2 (tistory.com)