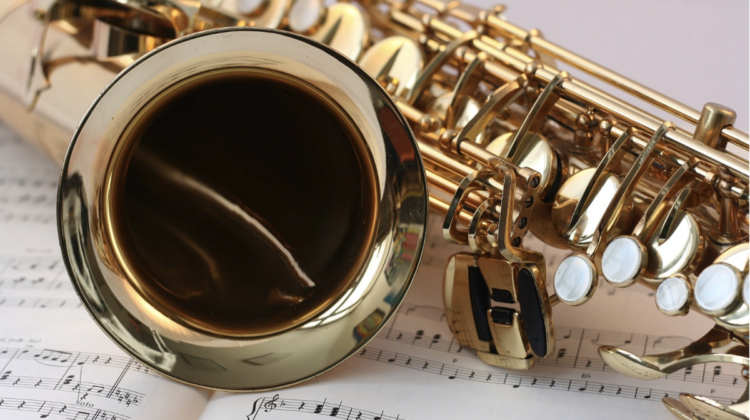
Let’s see how regularization and the learning rate alpha affect model performance.
As you’ll see shortly, tuning of hyperparameters affect a model’s accuracy and F1 score. Not sure what these metrics mean? See their definitions in my previous Titanic article.
Effect of regularization
I used SciKit-Learn’s LogisticRegression classifier to fit and test my data. There are many solvers to choose from, each solver has their own algorithm for convergence. For illustrative purposes, I choose the “saga” solver. It’s the only solver to support L1, L2, and no regularization.
Note: for Scikit-Learn’s LogisticRegression, instead of the λ regularization parameter, the classifier takes in a “C”, which is the inverse of regularization strength. Think of it as 1/λ.
I used SciKit-Learn’s GridSearchCV to obtain the model’s score for every combination of penalty = ["none", "l1", "l2"]
and C = [0.05, 0.1, 0.5, 1, 5]
.
from sklearn.model_selection import GridSearchCVclf = LogisticRegression(solver='saga', max_iter=5000, random_state=0)param_grid = { 'penalty': ['none', 'l1', 'l2'], 'C': [0.05, 0.1, 0.5, 1, 5] }grid_search = GridSearchCV(clf, param_grid=param_grid)grid_search.fit(X, y)result = grid_search.cv_results_
GridSearchCV does an internal 5-fold cross validation. The average of model scores for each combination is:
L2 regularization with a C of 0.1 performed the best!
Side note #1: I also implemented a random search algorithm with SciKit-Learn’s RandomizedSearchCV. If you’re curious, you can find the example in my Jupyter Notebook.
Side note #2: I’m sure you noticed that no regularization performed better than L1, and in many cases, there was no difference between no regularization and L2. The best explanation I have is that SciKit Learn’s LogisticRegression might already be working well without regularization. Nevertheless, regularization did bring some improvement.
We’ll see later that regularization does play a big role in the SGDClassifier.
I then did a side-by-side comparison of several performance metrics without regularization and with L2 regularization.
tuned = LogisticRegression(solver='saga', penalty='l2', C=0.1, max_iter=5000, random_state=2)not_tuned = LogisticRegression(solver='saga', penalty='none', max_iter=5000, random_state=2)tuned.fit(X_train, y_train)
not_tuned.fit(X_train, y_train)y_pred_tuned = tuned.predict(X_test)
y_pred_not_tuned = not_tuned.predict(X_test)data = {
'accuracy': [accuracy_score(y_test, y_pred_tuned), accuracy_score(y_test, y_pred_not_tuned)],
'precision': [precision_score(y_test, y_pred_tuned), precision_score(y_test, y_pred_not_tuned)],
'recall': [recall_score(y_test, y_pred_tuned), recall_score(y_test, y_pred_not_tuned)],
'f1 score': [f1_score(y_test, y_pred_tuned), f1_score(y_test, y_pred_not_tuned)]
}pd.DataFrame.from_dict(data, orient='index', columns=['tuned', 'not tuned'])
Tuned performed better than not tuned in every metric except recall. Again, read this blog post if you need a refresher on what these metrics mean.
Effect of learning rate (and regularization)
To see how different learning rates can affect model performance, I used SciKit Learn’s SGDClassifier (stochastic gradient descent classifier). It allows me to tweak learning rate whereas the LogisticRegression classifier does not.
There are three parameters to SGDClassifier we could tweak: alpha
, learning_rate
, and eta0
. The terminology is a bit confusing, so bear with me.
The learning_rate
is the type of learning rate (“optimal” vs. “constant”).
The eta0
is the algorithm’s learning rate when learning_rate
is “constant”. Normally, I call eta0
alpha.
The alpha
is the constant that multiplies the regularization term. It’s also used to calculate the learning rate when learning_rate
is “optimal”. alpha
serves the purpose of what’s commonly referred to as lambda.
Thus, there are several ways to set learning rate in SGDClassifier. If you want a constant learning rate, set learning_rate='constant'
and eta0=the_learning_rate_you_want
. If you want a dynamic learning rate (that depends on the step you’re at), set learning_rate='optimal'
. In the case of “optimal”, eta0
is not used, and alpha
serves the dual purpose of regularization strength and a constant in computing the dynamic learning rate at each step.
Below is a grid search algorithm for finding the best hyperparameters (for constant learning rate). I’m using the “constant” learning rate and I set the maximum iteration to 50,000.
from sklearn.linear_model import SGDClassifier
import matplotlib.pyplot as pltsgd = SGDClassifier(loss="log", penalty="l2", max_iter=50000, random_state=100)param_grid = {
'eta0': [0.00001, 0.0001, 0.001, 0.01, 0.1, 1],
'learning_rate': ['constant'],
'alpha': [0.00001, 0.0001, 0.001, 0.01, 0.1, 1]
}grid_search = GridSearchCV(sgd, param_grid=param_grid)grid_search.fit(X, y)result = grid_search.cv_results_
The searcher gave alpha
(here it means regularization strength) of 0.1 and eta0
(learning rate) of 0.0001 as the best params with a score of 0.7176.
I’ve plotted accuracy vs. learning rate (eta0
) for couple different values of regularization strength (alpha
). You can see that learning rate as well as regularization strength both have significant effect on a model’s performance.
The accuracy is pretty low for 0.00001 learning rate. This is likely due to the algorithm converging too slowly during gradient descent; after 50000 iterations, we’re nowhere near the minimum. The accuracy is also low for high learning rate (0.1 & 1). This is likely due to overshooting. Below is a more scaled plot with all the alphas.
Regularization strength (alpha) plays a role in accuracy too. For any given learning rate (eta0), there’s a large distribution of accuracy based on what the alpha value is.
Learning rate and regularization are just two hyperparameters in machine learning models. Every machine learning algorithm have their own set of hyperparameters. Questions? Comments? Respond below.