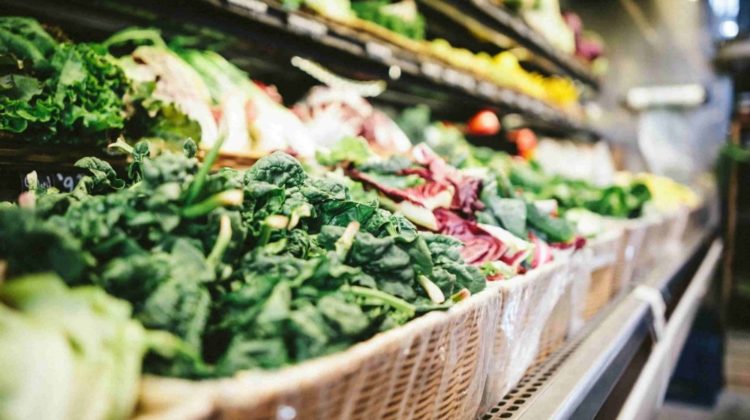

A staggering 600 million people –which is almost 1 in 10 people in the world, became ill after ingesting contaminated food. Of those, 420,000 people die every single year. Just alone in America, there are 48 million cases of foodborne illnesses annually — tantamount to 1 in 6 Americans getting sick every year.
Conceptualize a world where your basic needs like food can get you sick. Many don’t even need to conceptualize because it is their reality.
So what’s the solution?
Hyperspectral Imaging.
“It collects hundreds of images at different wavelengths for the same spatial area. While the human eye has only three color receptors in the blue, green and red, hyperspectral imaging measures the continuous spectrum of the light for each pixel of the scene with fine wavelength resolution, not only in the visible but also in the near-infrared.” — Nireos
Don’t get me wrong, our eyes and mobile cameras surely do an amazing job of capturing colourful and vivid art pieces. But, there are still limitations to what our human eyes can perceive and details our RGB cameras can capture. Here’s when Hyperspectral Imaging enters the game. Hyperspectral Imaging is an analytical technique that is based on spectroscopy.
It analyzes a broad spectrum of light rather than assigning primary colours (red, green, blue) to each pixel.
Essentially the goal of this form of imaging is to obtain the spectrum for each pixel in the image. This allows us to find objects, detect processes, identify materials we couldn’t have with our conventional microscopes, cameras or our very eyes.
How does this tie in with food?
Well, hyperspectral imaging can be implemented to detect foodborne pathogens. Traditional methods for the detection and identification of pathogens are merely cumbersome. In the picture below, you can see pathogens such as E. coli and Salmonella being detected.
Not only can hyperspectral imaging be used for food safety but also for the assessment of food quality. It allows us to determine the freshness of meat, vegetable, and fruit products. Which in turn, accurately calculates the shelf life of the products.
Hyperspectral imaging is also used in other industries such as precision agriculture, biotechnology, environmental monitoring and much more.
But… There’s a HUGE but …
The hyperspectral cameras (aka image spectrometer) ARE SOO EXPENSIVE! The cost ranges from $28k to well over $1M! Fortunately, scientists have been working on ways to lower the cost of these cameras to around $800. However, there is still another way to produce hyperspectral images using …
Artificial Intelligence.
Artificial Intelligence x Hyperspectral Imaging
There are several ways you can produce hyperspectral images from AI. In this article, I am going to focus on generative adversarial networks (GAN) based on the Pix2Pix model as they are computationally less expensive and has a lower training/validation loss than the other methods.
The Pix2Pix GAN is an approach to train a deep convolutional neural network for image-image conversions. Now in a GAN model, there is a generator and a discriminator.
The role of the generator is to generate a hyperspectral image from a ‘random input’ which is the RGB image. Once the ‘fake’ hyperspectral image is created, it runs through the discriminator.
There are two inputs to the discriminator: A real hyperspectral image and the generated hyperspectral image. The role of the discriminator is to discriminate between which image is the ‘real’ hyperspectral image.
A simple way to think about generative adversarial networks would be …
Let’s say Bob (the generator) is pretty broke so he decided to start making counterfeit money. He goes to the store and tries to pay with his counterfeit bill. Now the cashier (the discriminator), can differentiate between Bob’s counterfeit and a real bill. The cashier declines the bill.
So Bob tried again. And got rejected.
He tried again and got rejected 100 times. At this point, both the generator (Bob) and the discriminator (the cashier) are getting better and better at making fake bills and differentiating bills.
Finally, Bob becomes very good at making money and tricks the cashier.
Success!
The same notion is applied to creating hyperspectral images. The generator creates the images and the discriminator receives two inputs: the actual hyperspectral image taken on a hyperspectral camera, and the generated hyperspectral image. Both of the networks get trained. The generator gets better and better at making hyperspectral images.
There are a lot of different applications for this such as checking if a fruit is ripe or how long can you leave it on your shelf before it rots. Hopefully, in the future, this can be implemented to prevent deaths and hospitalization from food-borne diseases.
Summary
- You can use hyperspectral images to assess food quality, freshness, and if it was ever contaminated using hyperspectral imaging
- Hyperspectral imaging: An analytical technique that is based on spectroscopy
- Hyperspectral cameras are expensive, so a possible cost-effective way to make these images would be through artificial intelligence
- There are different way to make this AI, one is through generative adversarial networks (GAN)
If you liked this article be sure to give it a few 👏s and follow me on medium 🙂 Also, feel free to connect with me on LinkedIn
More resources!