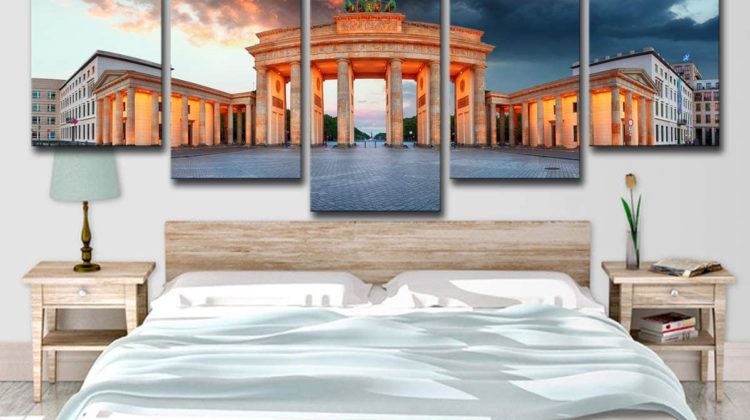
Prices can be very obscure. Hosts themselves may struggle to find a fair price. In return, guests sometimes disapprove and let them know in either good or bad reviews. This is a valid reason to investigate what hides behind the price. There is a variety of reasons but we have only 94 features (columns) for each listing. The 95th feature is the price itself. Let´s see how they correlate.
Correlation analysis
The word correlation says it all. It is a mathematical operation that measures the degree to which 2 variables move in relation to each other. The use of this technique supposes that we work with numerical data, therefore we have to get rid of categorical data and impute missing values when possible. After clean up, we end up with 43 features only.
The pairwise correlation of features across all listings yields a matrix known as the correlation matrix. The matrix is symmetrical around the diagonal. Each cell in the table shows the correlation between two variables. In the heatmap representation, the color is tuned by the magnitude of correlation.
The darker, the more correlated. The diagonal is so dark because it depicts the correlation coefficient of a feature with itself. It can’t be any better. Other features like reviews score are related to each other because they turn dark when they meet.
We are interested in the line corresponding to the price as ordinate. Along that line, we look for the darkest squares.
It is not so clear so we transpose the line into a table and sort it by the coefficients. We notice 3 distinct categories of correlation strength.
In pole position, alone, we find the number of people the home can accommodate. According to the second group, the price changes with the number of beds, bedrooms, and included guests. The third group lists the less correlated features. In there, the cancellation policy and the availability come first.
Correlation analysis reveals that price fluctuation is depending on practical features such as the capacity of the home, facilities, and cleaning fees.
Machine-learning analysis
There is another elegant way to understand what features contribute to pricing. The idea consists of building a machine learning model to predict the price based on all other given features. While training, the model should understand the underlying correlations between data and how they impact the price. Afterward, we will try to identify what features are the most relevant to him.
For this regression problem, we use an ensemble of decision trees optimized with a gradient boost regressor. The model achieves to predict the price with a 5$ error margin on training data (17606 inputs ) against 13.5$ on tests data (4402 inputs). The error is still quite high but we consider it is good enough to know what features matter. How to? We must score feature importance first.
2 methods:
- Open: Decision tree models are explicit. Feature importance score is inherent to the learning process of decision trees. It is used to know what features are important for branching.
- Closed: It deals with the model as a black box. This method is known as the permutation method because of how it works. The model tries to make predictions while the values of one feature are swapped repeatedly. If the predictions are completely wrong, then the model is mostly relying on that feature, otherwise, it is not.
In both cases the higher is the score, the more important is the feature. Let’s have a look at the top 15:
Both methods agree on the 2 topmost important features in price prediction: The room types and the number of people it can accommodate. So far It fits with the correlation analysis.
The first method grants particular importance to the position (latitude and longitude). It makes sense but this is something new. For the following features, the model picked up the most correlated ones. Again, we find bedrooms, cleaning fees, and included guests.
Compared to the first method, the second one confirms the importance of the same features but not in the same order. Instead of position, we find cleaning fees, extra people’s costs, and minimum nights stay.
In machine-learning and correlation analysis, availability features are not very important but recurrent. They (30, 60, 90, 365) are strongly correlated to each other so their influence on the price is comparable. They should be considered as a whole. It seems that the concept behind that variable has an impact on the price.
The price depends on the room type first, and then on the home capacity. These two features are undeniable. Facilities and cleaning fees are significant, extra people and positon are noteworthy. The impact of availability is not well established but it is worth further investigation. Surprisingly, the review scores are missing.