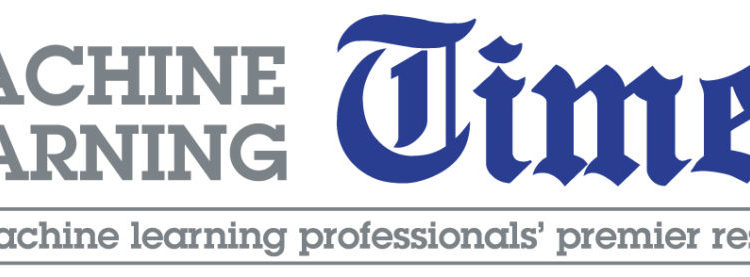
Originally published in UX Collective, Jan 19, 2021.
For an industry that prides itself on moving fast, the tech community has been remarkably slow to adapt to the differences of designing with AI. Machine learning is an intrinsically fuzzy science, yet when it inevitably returns unpredictable results, we tend to react like it’s a puzzle to be solved; believing that with enough algorithmic brilliance, we can eventually fit all the pieces into place and render something approaching objective truth. But objectivity and truth are often far afield from the true promise of AI, as we’ll soon discuss.
I think a lot of the confusion stems from language; in particular the way we talk about “machine-like efficiency”. Machines are expected to make precise measurements about whatever they’re pointed at; to produce “data”.
But machine learning doesn’t produce data. Machine learning produces predictions about how observations in the present overlap with patterns from the past. In this way, it’s literally an inversion of the classic if-this-then-that logic that’s driven conventional software development for so long. My colleague Rick Barraza has a great way of describing the distinction:
- In traditional programming, people input *rules* that guide how the system produces *outcomes*.
- In machine learning, people input *outcomes* that guide how the system produces *rules*.
To continue reading this article, click here.