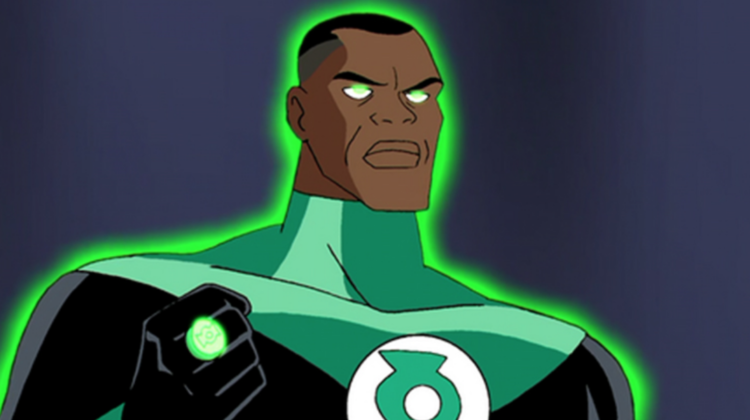

Unlike Carmen San Diego or Atlantis, finding viable datasets is not hard if you know where to look. Since the recent explosion in interest and application of Deep Learning, datasets have become the gas to power the ever growing monstrous machine learning model of modernity. From the shows you watch on Netflix, the steps that you struggle to make on your Fitbit, or the photos you like on Instagram and Pinterest, all of the data are collected, cleaned, and processed to provide you, the quintessential patron of the Internet of things, a relatively seamless and customized user experience. Data is like gold except its useful is not intuitive. A dataset is a collection of data organized in rows and columns and stored in a CSV file. A Comma-Separated Value file with thousands of rows and columns has little to no use for those who do not know how to speak, or script, the magic words to elicit great riches. Therefore data is less like gold and more similar to Green Lantern’s ring.
Green Lantern’s ring only worked when the right words were spoken.
“In brightest day, in blackest night, no evil shall escape my sight. Let those who worship evil’s might, beware my power, Green Lantern’s light.”
Afterwards, Green Lanterns were only limited to the power of their imagination. Of course it takes more than just some fancy pansy poem to solicit supreme power from our datasets, but the concept is the same. I’m being reductive for the sake of brevity, but find the data, recite a haiku or two in script, and out pops valuable information. So, where do we find the data?
Before I show you to the El Dorado of data it is important to make sure that what we are using to train our models is in fact quality data. It would suck to travel miles to the fountain of youth just to find out it is only Flint, Michigan tap water. Finding and maintaining quality data is essential in Data Science and Machine Learning. The more inaccurate or biased the data is that your model is trained on, the more inaccurate or biased your model’s predictions will be.
An articled on Blazent “Seven Characteristics That Define Quality Data” written by Dan Ortega is a great article to start with when accessing the quality of your data. In case you are not into reading amazing articles, which would be impossible because you are here, I will list the seven characteristics Ortega enumerates below:
- Accuracy and Precision: Exactness and free of erroneous elements
- Legitimacy and Validity: Data outside of the boundaries of requirements — this can happen if you are referencing a poll and the creator offered “other” as an option in any of the questions
3. Reliability and Consistency: Ensuring consistent information across multiple sources
4. Timeliness and Relevance: Data collected at the wrong time can lose its significance — housing in San Francisco in 90s would not be useful gauging the housing market prior to Google
5. Completeness and Comprehensiveness: Holes in the data ultimately giving you a partial picture
6. Availability and Accessibility: This is what this article is all about — Free and accessible places to get data
7. Granularity and Uniqueness: How much detail is in your data?
Now that we understand what good quality data looks like, we can’t be fooled if we get to El Dorado and there is no real gold.
There are tons of places offering quality data for public use, but for the sake of brevity and for keeping our RDQ to a less than a ten minute read we will only list five:
Uhhhh duh! With the current goal becoming a Kaggle Grandmaster did you think we would start anywhere else? Through Kaggle you have access to tons of data curated by both users and reputable entities. I used Kaggle to get access to COVID-19 data early in the pandemic.
Google Public Datasets is flooded with big data. Whether you are planning to use the data to build machine learning models or just to find the devil in the details, Google provides massive amounts of data on a variety of categories. Just a warning; you will have to sign up but the first Terabyte of data is free so run free.
Five Thirty Eight is really dope. I love them because they have datasets that can be difficult to obtain without doing all of the web scraping yourself. You can find everything from data collected about Donald Trump’s Twitter to police killings. Their data is available through Github.
4. Data.world
I couldn’t make a list without Data.world. Data.world puts massive amounts of data at your fingertips. If the Green Lantern’s ring differed from person to person then Data.world would be that head Green Lantern person’s ring; I can not remember their name.
5. Quandl
See if you can pronounce the name without research. I had to include a platform for economic and financial data. Finance data is truly as close to gold as we are going to gold. Quandl is a NASDAQ platform that provides a variety of financial datasets. Not every dataset is free, but Quandl still provides more than enough to make our list.
Facebook, Netflix, Amazon, and Google all allow you to download your personal data. Check it out and see what devil you can pull form those details. Maybe you can find habits that you weren’t aware of. I have pulled my location data from Google and I am beginning to make a map of all the places I have visited. Plus, you will be extremely surprised and maybe even a bit creeped out about the amount of data they have on you.
It is important to remember that accurate and reliable data is essential to training accurate models. With the variety of data platforms available, you can pretty much ensure no evil will escape your sight, Green Lantern. The amount of possibilities will only be limited by your imagination. Go see what you can cook up by looking at these platforms and downloading your own data. Maybe you can join two or more datasets and find some Rosetta Stone level revelations.