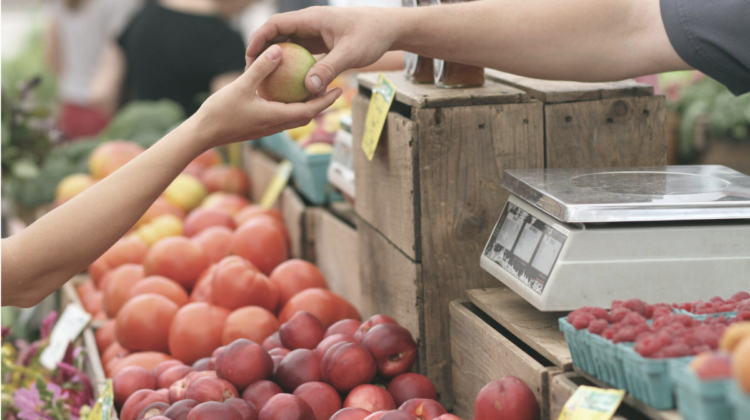
Episode 1: Teaching the AI to train itself

This is the first part of a three part series looking at why retailers struggle to adopt AI technology, what can be done to help them and why it’s important for tech companies to focus on making the processes easier, while directing all their efforts to improving business outputs.
Artificial Intelligence (AI) isn’t a new concept: for years, Hollywood has been throwing the name around to make its sci-fi blockbusters more futuristic. Now AI’s application has firmly found itself in the real world, and recently seems to be making a huge impact in many industries. This is due to the potential capabilities of it being able to speed up processes, lower the chance of errors occurring; and it’s more secure than a human in certain situations, to name but a few of the benefits.
Despite all these added benefits of adopting AI technology in businesses, there are still some industries lagging behind this curve. One of the slowest industries to adopt AI solutions is the ancient grocery retail industry. It is not a surprise to hear the grocery retail industry is lagging behind on the AI train but here are some reasons I believe are holding them back, but also some things they can do to catch up!
Why is there an adoption problem
NRF 2020 showed that there is an appetite to adopt AI into grocery retailers checkout systems, and with there being a vast number of companies offering possible solutions to retailers’ pain points such as preventing marginal loss at the checkout between similar items, identifying non-barcoded items with competent accuracy and also with Amazon Go making a massive push up the retail ladder, you’d expect most retailers would move with a bit more pace and already integrate some form of AI into their storefront. This just isn’t the case though.
The benefits grocery retailers could reap, such as reducing loss at the checkout, speeding up queues and order fulfilment processes, and improving customer experience, should be incentives enough to digitally transform. So clearly, there must be some other form of ‘issue’ that’s stopping them.
Lack of internal expertise and fear of complexity
For AI to be used by anyone, somebody has to train an AI Model that knows how to do what the customer needs it to do. To keep in line with the simple AI use case we were discussing earlier (the one that has been the cornerstone of NRF 2020), we shall focus on an Item Recognition Model that needs to identify the items placed on the checkout counter or scale. The main problem lies in the fact that these AI models need to be constantly retrained once they have been integrated into a store. Retraining is an inherent part of AI as Models always lose their accuracy and performance over time (in scientific terms this is called “Data Drift”). Most grocery retailers feel they don’t have the expertise, time or perhaps budget to adequately do that and also, this retraining process is usually why the AI software doesn’t work very well to begin with. If there is one thing that grocery retailers can’t afford is a missed prediction because then the AI is practically begging the customer to lie. This concern is not without merit, as adding a new or different looking product to an existing AI model usually requires some heavy duty tech work.
The issue with the AI model
Before I can discuss how this issue can be solved, we have to understand what the status quo is.
To do that, let’s look at the issues with the AI itself. In order for a retailer to reap the rewards of having AI technology at its Point of Sale systems, the prediction capability of the AI has to be competent — ultimately it needs to be as close to 100% accuracy as possible, and be able to easily identify non-barcoded items at the checkout.
To do this, PoS system AI are generally trained on the items already found in the store. This data is then stored and called upon each time that item comes up. For example, if we go into a supermarket, we can generally always find a banana. A banana would be easy for any PoS system to identify because it is an in-house standard and saved in the AI system’s database. Now, say it is Halloween and all of a sudden the store has pumpkins in. These items might not have been included in the first training of the PoS system and so, when a customer tries to pass it through the checkout, if you’re lucky it will come up with an error but in most instances the AI will try to find the closest looking item it has in its database. In the case of someone placing a pumpkin on the counter for recognition, the AI software will look for a similar item in it’s database and display the item on the checkout screen, which will most likely be a persimmon fruit which is clearly cheaper than a pumpkin. Not only is this frustrating for the consumer as they are being made to lie or go back to the pick-list menu, but of course can be a very costly error for the retailer too.
Under these circumstances, a company will have two options in order to get over this issue: either retrain the AI itself or get a third-party provider to do so.
In both cases, the integrated AI solution will need to be retrained by the company’s own technicians, meaning they will have to prioritise this over any other scheduled tasks they have. Given the different conditions in each store of the same chain, there is a need to collect data from all different stores. This is done by scanning the item a couple of hundreds of times. A task that might sound simple, but is very time consuming. The process of collecting new data and uploading it to the cloud or server for training is not only time consuming, but can have huge costs for the retailer.
Using conventional methods to upload an exabyte of data (which is what Walmart generates in a week) will take all of 26 years to complete! And to combat this issue, Amazon have created the AWS snowmobile which is a truck that carries an exabyte of data faster than the internet.
When you consider that each new item that is brought into the store will have to go through this process, then it’s clear that these options are not cost effective. A lose-lose situation from the store’s point of view and a reason why adoption rates could be so low. Retraining used to be a valid concern.